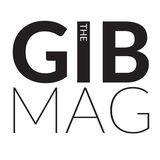
Share Public Profile
Rock Publishing Ltd
The Gibraltar Magazine is your monthly business, entertainment, and lifestyle source. Providing the community with the latest breaking news and quality content since 1995. Covering business, leisure and the social scene, there's something to read for everyone — including what's on, street map, restaurant guide and business directory. Contact: getintouch@thegibraltarmagazine.com